1. 富集分析(enrichment analysis)
富集分析(enrichment analysis)的概述参考博客:富集分析概述。
2. topGO
2.1. topGO简介
topGO是一个R包,用于半自动的GO terms的富集分析。
因为topGO只用于GO的富集分析,且是半自动化的,推荐使用更方便的在线工具KOBAS-i;KOBAS-i 备用;GOEAST; 或者功能更完善的clusterProfiler包,参考博客:clusterProfiler包。
2.2. GO term
GO term分为三大类:
- cellular component(CC)-细胞成分(其中基因产物位于细胞内部)
- molecular function(MF)-分子功能(基因产物的功能是什么)
- biology process(BP)-生物过程(即基因产物参与的一系列事件)
三类都可以用topGO做富集分析。
2.3. topGO支持的统计检验方法
topGO包默认算法用的是weight01,是elim和权重算法的混合。
topGO支持的统计方法 | fisher | ks | t | globaltest | sum |
---|---|---|---|---|---|
classic | Y | Y | Y | Y | Y |
elim | Y | Y | Y | Y | Y |
weight | Y | N | N | N | N |
weight01 | Y | Y | Y | Y | Y |
lea | Y | Y | Y | Y | Y |
parentchild | Y | N | N | N | N |
2.4. 安装topGO
1 | # 安装topGO软件包 |
或者
1 | source("https://bioconductor.org/biocLite.R") |
2.5. topGO做富集分析(ORA)
2.5.1. 输入文件
两个输入文件
- genes.list:需要做富集分析的geneID的list,一个基因ID一行
- sample.anno:基因及GO注释信息,第一列是geneID,第二列是GO注释,空格分隔,GO注释可以有多个,格式为GO:0000428,GO:0003677,GO:0005506,
2.5.2. 富集分析
1 | # 设置工作目录,后面读取文件什么的就可以直接读取不需要那么长的路径 |
2.5.3. 结果解释
- .xls结果文件中每一列的含义
GO.ID:富集的GO ID
Term:GO ID的描述
Annotated : number of genes in go.db which are annotated with the GO-term.在go.db中被注释到GO-term的基因数量。
Significant : number of genes belonging to your input which are annotated with the GO-term. GO-term被注释到的基因中包含输入的基因的数量
Expected : show an estimate of the number of genes a node of size Annotated would have if the significant genes were to be randomly selected from the gene universe. 对节点基因数量的预期
KS:用KS(Kolmogorov-Smirnov)算法计算得到的p-value值。
KS全称是:Kolmogorov-Smirnov,KS值是通过KS检验所得,KS检验是一种算法。统计方法如下:
- 首先计算每个go节点对应的gene个数,
- 如果某个节点的子节点也有gene比对上,那么父节点对应的gene个数也要加上子节点的基因数
- 使用KS统计检验进行p值的计算,文件中的KS值,就是常说的p value,叫KS值的原因,是体现使用的KS检验方法。
- DAG图(矢量图/像素图)
有向无环图(Directed acyclic graph, DAG)中,从上至下依次有包含关系,只有从上往下的箭头,这个箭头代表包含关系,即有向。只有从上往下的箭头,方向是确定的,所以不会形成闭环,即无环。

Figure 1. DAG图示例
from wikipedia:directed acyclic graph
- 有向无环图能直观展示基因富集的GO节点(Term)及其层级关系。
- 在有向无环图中,矩形代表富集到的top10个GO Terms,颜色从黄到红,对应p值从大到小。
- 分支代表包含关系,从上至下所定义的功能描述范围越来越具体。
- 箭头代表包含关系,即该节点的所有基因同样注释到其上级节点中。
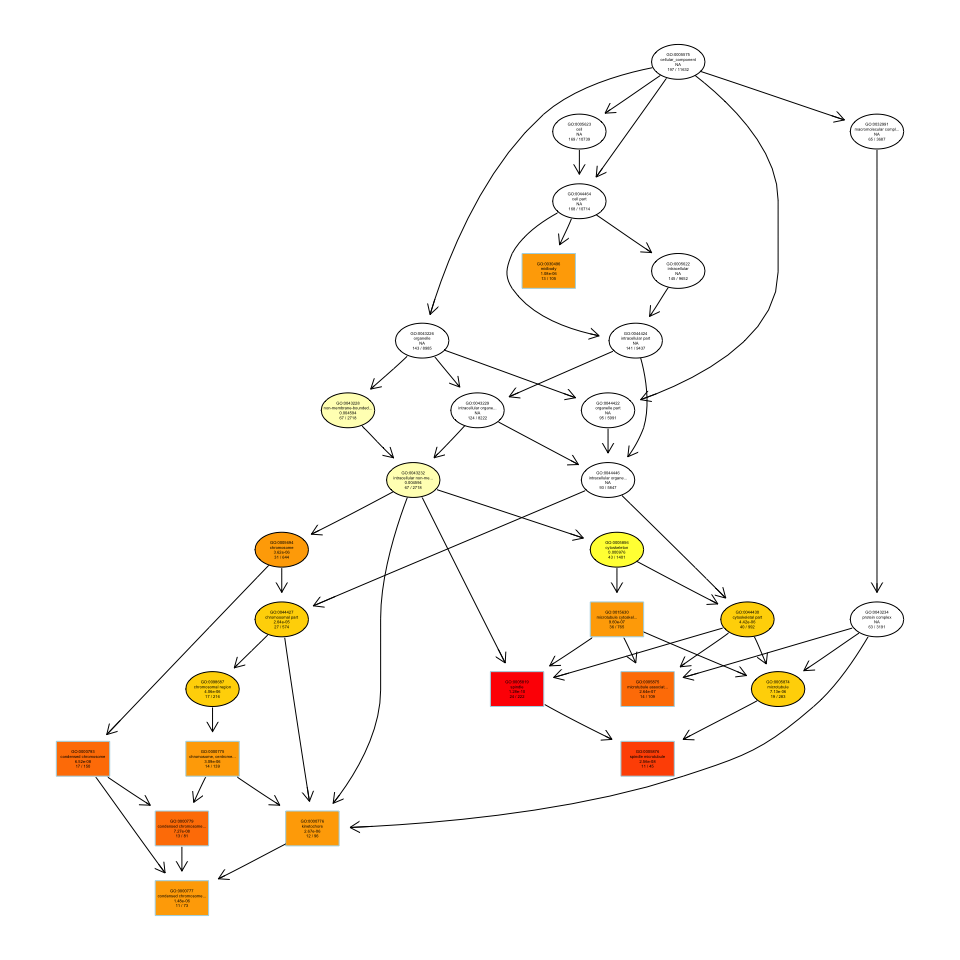
Figure 2. topGO结果DAG图
from clusterProfiler blog
注:对每个GO节点进行富集,在图中用方框表示显著度最高的10个节点,图中还包含其各层对应关系。每个方框(或椭圆)内给出了该GO节点的内容描述和富集显著性值。不同颜色代表不同的富集显著性,颜色越深,显著性越高。
3. references
- topGO tutorial:https://bioconductor.org/packages/release/bioc/vignettes/topGO/inst/doc/topGO.pdf
- topGO blog:https://datacatz.wordpress.com/2018/01/19/gene-set-enrichment-analysis-with-topgo-part-1/
- R topGO:https://www.codenong.com/cs105162324/
- 欢迎关注微信公众号:生信技工
- 公众号主要分享生信分析、生信软件、基因组学、转录组学、植物进化、生物学概念等相关内容,包括生物信息学工具的基本原理、操作步骤和学习心得。
